Interpreting Connections in Artificial Neural Networks
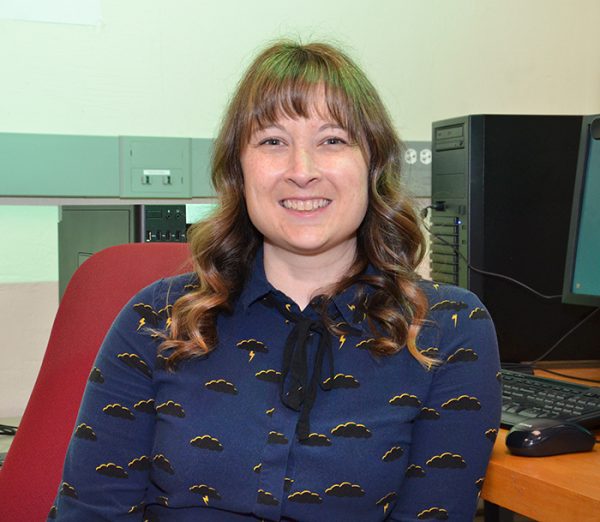
Dr. Stephanie Pilkington and her PhDisaster research group within the Civil Engineering Technology and Construction Management group focus their research on natural hazards, the impact to communities, and the subsequent recovery process. This can range from infrastructure damage assessments and modeling, to understanding how communities, infrastructure and environment interact. The research is trans-disciplinary in nature and incorporates aspects from civil engineering, computer science, atmospheric and earth science, social science, economics, and policy and management.
In a recent project, Dr. Stephanie Pilkington decided to interpret the neural connections made in an Artificial Neural Networks (ANN) using graph theory.
Research Highlight
Artificial Intelligence (AI) and machine learning have been growing in popularity as predictive modeling tools. However, some criticism remains that there is a “black box” nature to machine learning models. Specifically, for Artificial Neural Networks (ANNs), input and output data are provided to a training algorithm, which goes through a series of iterations to determine patterns that relate those inputs to outputs. From a modeling standpoint, it would appear that data is “fed” into the model without much understanding of how the resulting outputs are reached even if modeling errors are low overall. Other modeling approaches are often used to “explain” the resulting ANN model. In contrast to this approach, Dr. Stephanie Pilkington and Dr. Hussam Mahmoud (Colorado State University) decided to interpret the neural connections made in an ANN using graph theory.
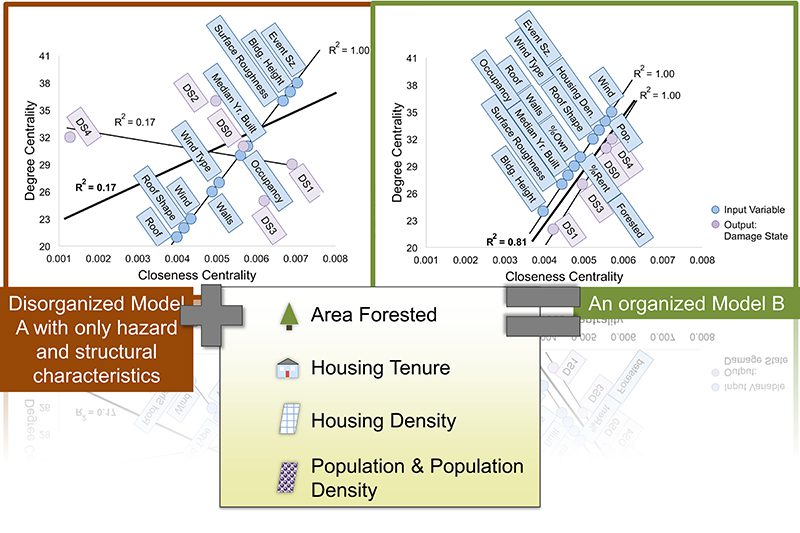
Drs. Pilkington and Mahmoud, both with expertise in community resilience from natural hazards, decided to combine social demographics of a neighborhood with building structural characteristics to predict the resulting level of damage (damage state) from an extreme wind hazard event using ANNs. Models with social demographic variables and without were created and interpreted by tracking patterns through neuron connections using graph theory. This approach to interpreting an ANN was inspired from neurological studies conducted to better understand brain patterns in individuals with schizophrenia and Alzheimer’s. Using this approach, it was found that when social factors are included in predicting building damage due to extreme wind, the ANN connections shift and ultimately show an importance of how a building is used, not just it’s material and structural configuration, as is commonly used in modeling damage. These findings have recently been published by Royal Society Open Science at https://royalsocietypublishing.org/doi/10.1098/rsos.200922 .
Drs. Pilkington and Mahmoud have conducted a similar analysis on a community recovery from wind hazards ANN model. The findings from building and analyzing this model are currently being compiled.
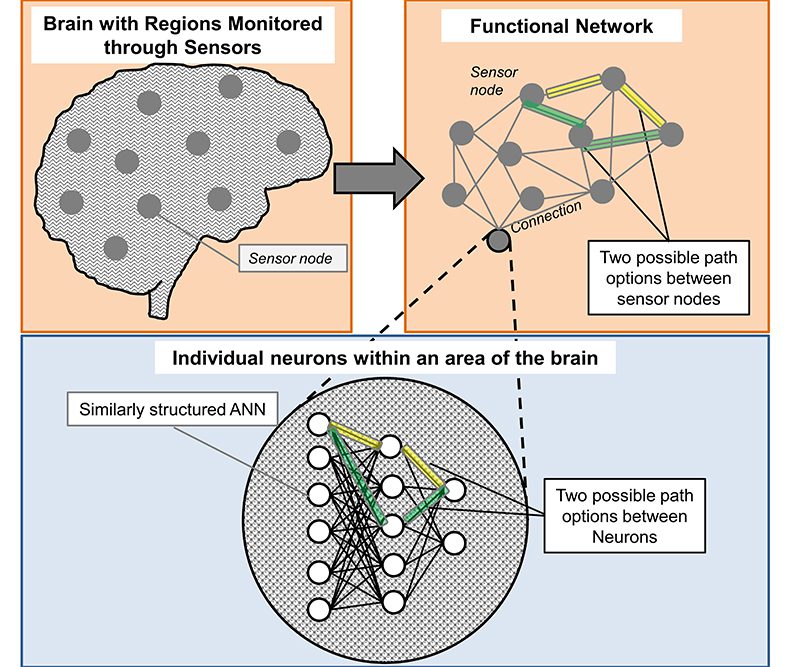